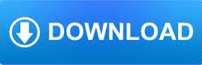
Here we take "mpg" as the response variable, "hp" as the predictor variable and "am" as the categorical variable. Input DataĬreate a data frame containing the fields "mpg", "hp" and "am" from the data set mtcars. It is done by using the aov() function followed by the anova() function to compare the multiple regressions. We study the effect of the value of "am" on the regression between "mpg" and "hp". The miles per gallon value(mpg) of a car can also depend on it besides the value of horse power("hp"). It is a categorical variable with values 0 and 1. In it we observer that the field "am" represents the type of transmission (auto or manual). ExampleĬonsider the R built in data set mtcars. Such an analysis is termed as Analysis of Covariance also called as ANCOVA. In such scenario, we can study the effect of the categorical variable by using it along with the predictor variable and comparing the regression lines for each level of the categorical variable. The simple regression analysis gives multiple results for each value of the categorical variable. Sometimes, if we have a categorical variable with values like Yes/No or Male/Female etc. The first few lines of the phenotypic dataset $\\texttt$), and their precision will depend on the amount of information a parent has (_i.e.We use Regression analysis to create models which describe the effect of variation in predictor variables on the response variable. We also have pedigree information for the 43 parents. In this dataset, each family is formed by between 1 and 16 individuals (with an average of 12.2). There were no reciprocal or self-pollinated crosses planned, but these can occur in other crops and they might need to be identified and modelled properly. In this dataset we have a total of 71 families that originated from 43 parents however, 20 of those parents were used as both males and females in different crosses. We will be using the adjusted mean values for this trait.
#Asreml r aliased intereaction full#
A subset of the full dataset, corresponding to diameter at breast height (DBH, inches) measured at 6 years since planting at the Nassau (Florida, USA) site, is used here.
#Asreml r aliased intereaction series#
Individuals from these families were vegetatively propagated (cloned) and established in a series of field trials. Parents were crossed in a circular mating design, constituting several full-sib families. The data used here originates from a loblolly pine clonal study published by Resende _et al._ (2012). We will illustrate this here using an example of loblolly pine (_Pinus taeda_) in which some individuals, depending on the availability of pollen or flowers, were used in several artificial crosses as both male and female in the breeding program. This is done by _overlaying_ design matrices of the factors associated with male and female parents. In quantitative genetic analyses, monoecious species present a particular challenge, as a given parent can contribute to the estimation of its breeding value (or GCA) as both a male and a female, something that needs to be taken into consideration when a statistical model is fitted. Some examples of monoecious species are corn, squash, banana, and many conifers, particularly those of the genus _Pinus_. If there is an MD5 file, you should probably delete it. In contrast, dioecious species have distinctive male and female plants. Place NAMESPACE in the top folder of the unzipped package (should be something like asreml.d/ there will also be a DESCRIPTION file there). However, several commercial plant species are monoecious, which means that a given genotype will bear both male and female flowers. In most cases it is easy to assign the sex of a given individual. In many plant breeding programs, a parent is considered in several crosses. These BLUPs, which are the _general combining ability_ (GCA), or 1/2 of the _breeding value_ (BV, with BV = 2 ×× GCA) of each parent, are then used to select the best parents for future crosses or operational deployment. The progeny are later evaluated in a field experiment, and this information is used to assess the genetic worth of the parents by fitting parental linear mixed models (LMMs) and obtaining best linear unbiased predictions (BLUPs). Most breeding programs plan several controlled crosses between outstanding parents to detect favorable alleles in their offspring.
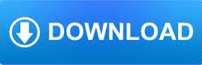